Artificial Intelligence & Machine Learning,
Next-Generation Technologies & Secure Development
Microsoft’s BitNet b1.58 2B4T: A Leap Forward in AI Efficiency
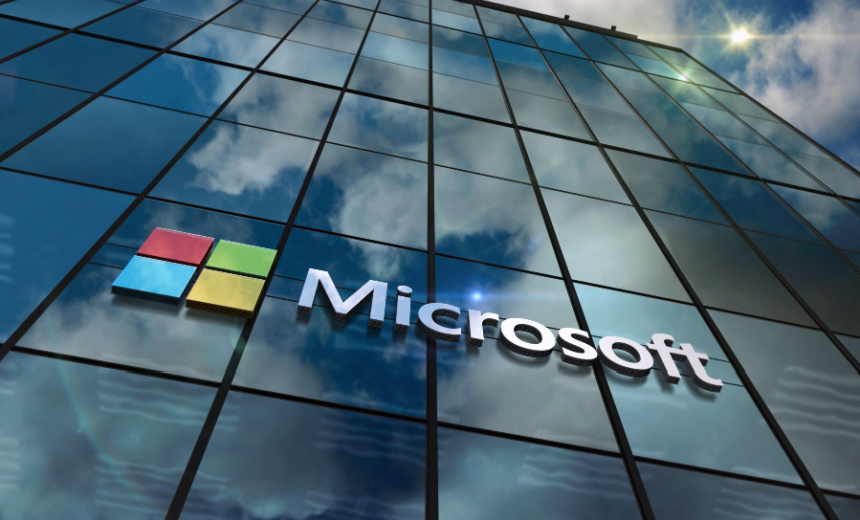
Microsoft has unveiled what it claims to be the most extensive 1-bit AI model to date, named BitNet b1.58 2B4T. This innovative model is designed to optimize speed and efficiency while enhancing accessibility to artificial intelligence technology.
In contrast to traditional large language models that heavily rely on GPUs and extensive infrastructure, BitNet is engineered to function effectively on CPUs, including Apple’s M2 chip. According to Microsoft researchers, the model is released under the permissive MIT license, signaling a shift towards more energy-efficient AI systems that are easier to deploy.
BitNet achieves its lightweight architecture by employing a simple quantization approach. Rather than utilizing full-precision or multi-bit quantization for model weights—key parameters that dictate a model’s functioning—BitNet employs three discrete values: -1, 0, and 1. This methodological simplification significantly reduces computational and memory demands, rendering it well-suited for devices with limited resources.
With 2 billion parameters trained on a dataset comprising 4 trillion tokens—equivalent to the contents of approximately 33 million books—BitNet represents a significant advancement in AI capabilities. In benchmark assessments, the model has outperformed notable competitors like Meta’s Llama 3.2 1B, Google’s Gemma 3 1B, and Alibaba’s Qwen 2.5 1.5B. Such tests cover varied tasks, including GSM8K, which involves elementary math problems, and PIQA, which assesses fundamental physical reasoning skills.
However, the merits of BitNet extend beyond mere accuracy. Microsoft asserts that the model operates at an impressive speed, sometimes delivering performance that is double that of its rivals, while also utilizing considerably less memory. This combination of rapid processing and efficiency positions BitNet as an attractive option for environments constrained by power and processing limitations.
Despite its advantages, there are notable limitations to consider. The reported performance enhancements are contingent on the use of Microsoft’s proprietary inference framework, bitnet.cpp
. While this framework facilitates optimal runtime performance, its compatibility with hardware is not universal. Specifically, it currently lacks support for GPUs, which are the standard choice for training and deploying AI models.