New Research Exposes Weaknesses in Tokenizers Used by Major LLMs
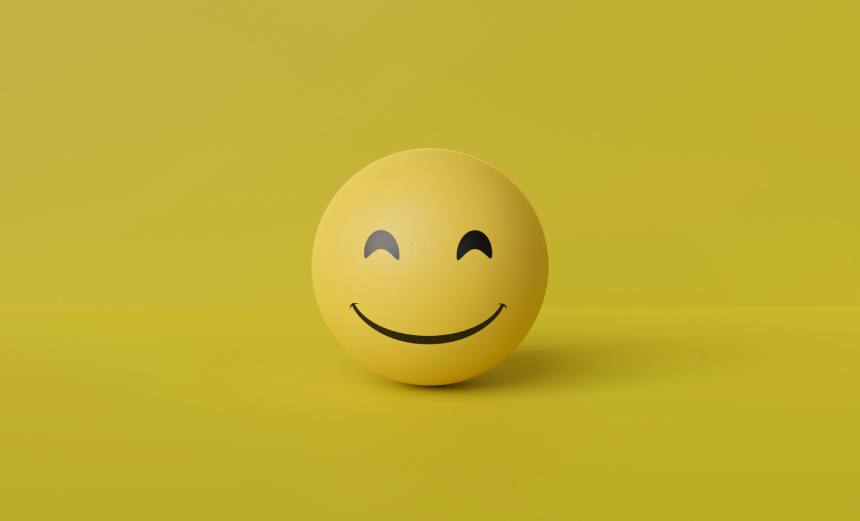
Recent findings indicate that sophisticated obfuscation tactics can effectively circumvent the safety mechanisms employed by today’s leading large language models (LLMs). A study led by Mindgard’s CEO, Peter Garraghan, demonstrated how adversaries exploit tokenizers to deliver malicious payloads using seemingly innocuous characters.
The research team evaluated LLM safety systems from several technology giants such as Microsoft, Nvidia, and Meta. Their findings reveal that even advanced security measures can be compromised through elementary methods, exposing vulnerabilities in the tokenization processes.
Differences in the efficacy of these models against evasion techniques are attributed to the training datasets utilized by the companies, particularly the quality of adversarial training designed to enhance model resilience. Garraghan highlighted that the current guardrail systems can be neutralized with minimal adjustments, mainly due to discrepancies between the tokenization views in these models.
The implications of these vulnerabilities are significant for critical sectors including finance and healthcare, where systems intended to protect generative AI can be deceived by slight modifications in text. This risk is heightened by the nature of tokenizers, which often misinterpret obfuscated content, leading to misclassifications of potentially harmful prompts.
Garraghan emphasized that existing guardrails are inadequate on their own and recommended a multi-layered strategy that begins with sanitizing inputs to eliminate suspicious characters. He proposed employing a combination of guardrails to analyze each prompt, identifying high-confidence threats while acknowledging the associated computational overhead and complexity.
This research not only sheds light on vulnerabilities in proprietary LLMs but also raises concerns about the potential for black-box systems, suggesting that attackers could leverage open-source implementations to enhance their strategies. The study points to an urgent need for increased understanding of attack methodologies and their implications for real-world security.
The use of invisible characters such as zero-width spaces and Unicode tags has emerged as a common technique for bypassing classification systems, maintaining visibility to LLMs while eluding detection. The challenge is compounded by the need for more robust classifier systems, which should incorporate runtime testing and behavior monitoring to identify evasion attempts proactively.
As the landscape of AI regulation develops, it is crucial that protective measures evolve accordingly, adopting best practices to bolster resilience against these threats. With AI technologies advancing towards multi-step processes, each additional layer presents new vulnerabilities that demand vigilant ongoing assessment in cybersecurity frameworks.