Artificial Intelligence & Machine Learning,
Next-Generation Technologies & Secure Development
Addressing Cybersecurity and Privacy Risks Associated with Autonomous Agents
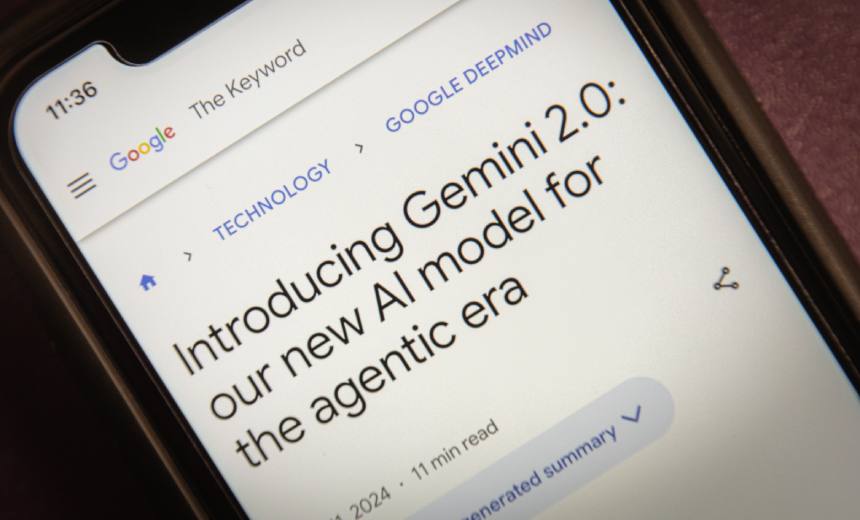
The adoption of artificial intelligence is accelerating as organizations integrate AI agents capable of executing complex tasks beyond standard automation. Tech companies are introducing autonomous AI tools designed to manage customer interactions, IT operations, and numerous operational processes. However, experts urge caution regarding the associated cybersecurity and privacy vulnerabilities that these advancements may invoke.
According to Avivah Litan, vice president and distinguished analyst at Gartner, AI agents excel in analyzing extensive datasets to uncover patterns and deliver actionable insights. These agents can facilitate customer service interactions, escalate issues, and even propose personalized solutions. In IT environments, AI agents have the potential to identify anomalies and initiate remediation measures, enabling businesses to enhance productivity while reallocating human resources towards strategic initiatives.
Despite their advantages, the deployment of AI agents introduces significant security challenges. Unlike traditional AI systems that function within predefined parameters, AI agents interact with diverse systems and external data streams, potentially increasing the risk of unauthorized access and data breaches. Litan highlights that previous issues, such as sensitive information leaks due to inadequate integration, and the possibility of malicious interference, could compel AI agents into executing unintended actions, resulting in operational disruptions or financial losses.
David Brauchler, CTO of cybersecurity firm NCC Group, elaborates on this risk by comparing traditional automation, which relies on predetermined rules, to AI agent systems dependent on data training and contextual instructions. The fundamental difference lies in the unpredictable nature of AI, which can complicate security outcomes and introduce security vulnerabilities that traditional automation methods do not face.
The interconnected and dynamic characteristics of AI agents present challenges for real-time threat detection, as they often outpace conventional security measures. Developers tend to underestimate that vulnerabilities can arise when these systems are connected to other environments, potentially amplifying the extent of the security risks involved.
Brauchler recommends establishing comprehensive risk assessment and threat modeling protocols for AI deployments, such as utilizing least-privilege access and dynamic capability shifts to enhance security safeguards. Effective architecture can help distinguish trusted from untrusted zones, thereby minimizing the risks of accidental breaches and enabling efficient anomaly monitoring through consistent scrutiny of agent activities.
Ensuring the security of AI agents hinges on a collaborative approach amongst developers, vendors, and users. This shared responsibility is essential in addressing potential vulnerabilities that, if overlooked, could lead to significant data breaches, financial repercussions, and reputational harm.
Finally, reinvigorating longstanding security architectures is essential to address the unique challenges that come with AI agents. Brauchler suggests adopting a trust-centered approach to data management instead of traditional patch-oriented strategies, as the convergence of data and code in AI necessitates a rethink of security infrastructures.