Artificial Intelligence & Machine Learning,
Next-Generation Technologies & Secure Development
The Leaderboard Landscape: More Advertising Than Authenticity
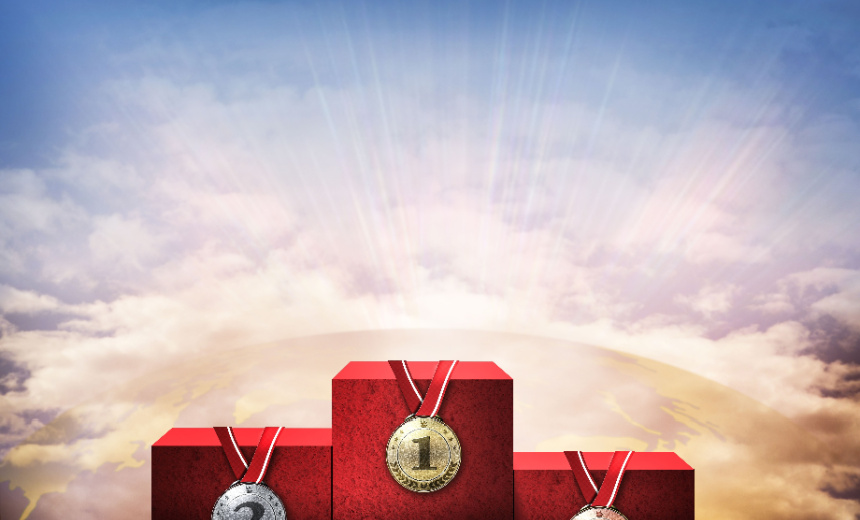
The publication of benchmark scores by artificial intelligence (AI) model developers is common practice, yet experts suggest that the competitive leaderboard may serve more as a promotional tool than a valid measure of AI capabilities. Reports by the European Commission’s Joint Research Center and Stanford University highlight potential flaws in the assessment methodologies used to evaluate these models.
Major players in AI, including OpenAI, Google, and Meta, have reported above-average scores on benchmarks of their own making. However, researchers warn that such results may be skewed due to factors like dataset contamination, biased testing methodologies, and simplistic task designs.
Stanford’s investigation of over 150 evaluation frameworks uncovered significant issues, such as data leakage, inadequate dataset diversity, and the misleading practice of score inflation via selective testing. Researchers expressed particular concern over tactics like “sandbagging,” which entails deliberately underperforming in specific tests to avoid scrutiny, drawing a parallel to the infamous Volkswagen emissions scandal.
Compounding these concerns, the European Commission identified systematic issues in AI benchmarking, including ambiguous dataset origins and tests that often fail to measure what they claim to. Their findings suggest that many benchmarks prioritize attracting investment over delivering meaningful evaluations, reinforcing outdated research approaches instead of adapting to the rapid evolution of AI technologies.
Stanford researchers emphasized the importance of understanding model failures over merely celebrating high scores, referencing past research that asserts the value of comprehensive evaluations. They argue that the reliability of benchmarking is crucial, especially as it underpins regulatory frameworks such as the EU AI Act, the U.K. Online Safety Act, and the U.S. AI Diffusion Framework.
Both reports advocate for a reassessment of AI benchmarks to ensure they meet the same standards of transparency and fairness expected of the models they are used to evaluate. Policymakers are urged to engage developers and organizations in discussing benchmark quality during AI evaluations and to adopt best practices for quality assurance.
The current landscape indicates a pressing need for more reliable benchmarking as regulation increasingly relies on these scores. With the understanding that “most benchmarks are highest quality at the design stage and lowest quality at the implementation stage,” there is an opportunity for significant improvement in how AI capabilities are assessed, potentially impacting future AI developments and their regulatory implications.