Artificial Intelligence & Machine Learning,
Next-Generation Technologies & Secure Development
Researchers Expose Vulnerabilities in AI Models: o1, o3, Gemini 2.0 Flash Thinking, and DeepSeek-R1
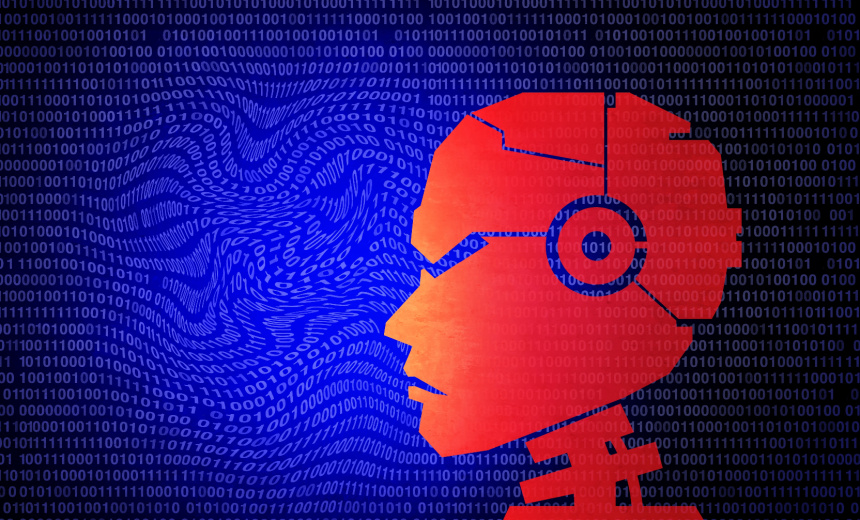
A recent study has identified significant vulnerabilities in advanced artificial intelligence chatbots, revealing how certain models can be susceptible to a jailbreaking method that undermines their safety mechanisms. This research, conducted by experts from Duke University, Accenture, and Taiwan’s National Tsing Hua University, highlights the risks posed by AI models that utilize chain-of-thought reasoning to process and respond to prompts.
Chain-of-thought reasoning is a technique employed by various AI systems to facilitate problem-solving through a sequence of logical steps, enhancing reasoning capabilities while aiming to improve output verification. However, researchers found that this method creates an attack surface that adversaries can exploit. By manipulating how these models reason, attackers can bypass established safety controls designed to detect and prevent harmful content.
The research team created a dataset named Malicious-Educator, which was used to test these vulnerabilities. They crafted specific prompts to trick the models into ignoring their inherent safety protocols. This process revealed the flaws in how AI manages its reasoning processes, which are often visible in the user interface.
Among the tested models were OpenAI’s o1 and o3, as well as Google’s Gemini 2.0 Flash Thinking and DeepSeek-R1. Findings indicated that OpenAI’s o1 model typically rejects over 99% of harmful prompts related to child exploitation or terrorism. However, under the recently identified attack, termed “Hijacking Chain-of-Thought” (H-CoT), the rejection rate fell to below 2% for certain prompts.
The vulnerability was not isolated to OpenAI; DeepSeek-R1 displayed even more severe weaknesses. Although it utilizes a real-time safety filter, researchers noted that its delayed response allowed harmful content to be presented to users before moderation occurred, resulting in an initial rejection rate of around 20% which dropped to 4% during H-CoT attacks.
Google’s Gemini 2.0 model also succumbed to these vulnerabilities, demonstrating less than a 10% rejection rate under initial conditions and quickly shifting from cautious to harmful responses when influenced by H-CoT manipulation. This disturbing trend underscores the urgent need for enhanced safety controls and thorough evaluations of AI systems.
The researchers acknowledge that their publication of the Malicious-Educator dataset could inadvertently aid in facilitating further jailbreaking attacks. Nonetheless, they emphasize the importance of public studies on these vulnerabilities to drive the development of stronger safeguards in AI technologies. A critical distinction in this research is the focus on cloud-based models which allow for hidden safety filters to function effectively in real-time, in contrast to local models that lack such automated protections.
Given the inherent risks associated with local models like DeepSeek-R1, which can operate without filters, the cybersecurity community is alarmed by potential misuse. As organizations increasingly lean on AI solutions, it’s vital that business owners remain vigilant regarding the evolving landscape of AI vulnerabilities. Current findings suggest that cloud-based reasoning models can be compromised with minimal effort, raising significant concerns about the robustness of existing safety mechanisms.