Finance & Banking,
Fraud Management & Cybercrime,
Fraud Risk Management
Enhancing Continuous User Authentication with Machine Learning and Generative AI
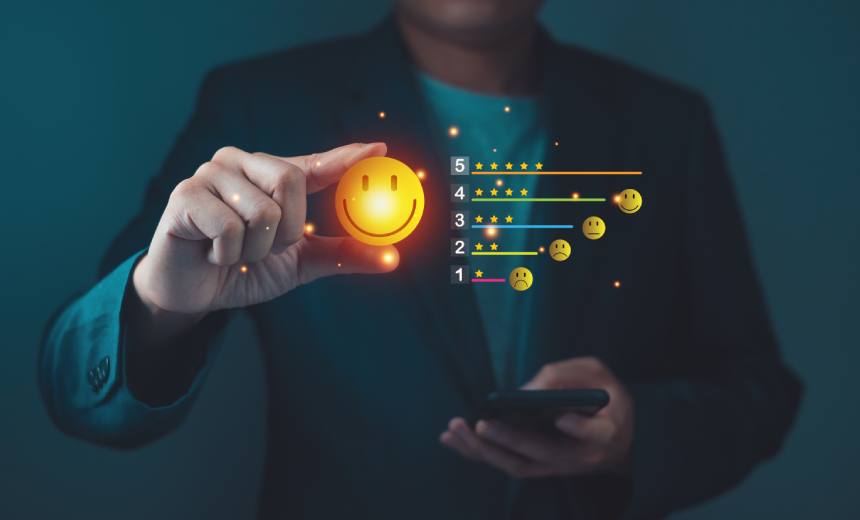
Recent advancements in artificial intelligence, particularly in behavioral biometrics, are becoming crucial countermeasures against identity theft orchestrated by cybercriminals through techniques such as deepfakes and synthetic sessions. Financial institutions are increasingly relying on AI-driven solutions that transcend traditional static credentials, providing real-time identity verification through ongoing analysis of user interactions. By monitoring actions like clicks, pauses, and keystrokes, these institutions are transforming one-time authentication methods into continuous identity assurance.
Jeremy London, the director of engineering for AI and threat analytics at Keeper Security, elaborates on the capabilities of modern AI systems that can assess thousands of behavioral data points to detect even the most subtle user patterns. This continuous comparison of live sessions with dynamic profiles significantly reduces the dependency on conventional password mechanisms and one-time multifactor authentication. In today’s financial services landscape, where simple stolen credentials are insufficient for effective fraud prevention, contextual machine learning could revolutionize authentication measures.
London explains, “AI-powered contextual intelligence enables continuous authentication by correlating user behavior with various environmental factors.” This adaptive methodology, aligned with the NIST principle of Continuous Multi-Factor Authentication, detects potential account takeovers without compromising the user experience. By validating identity in the background, financial institutions can reduce the friction often caused by traditional security measures.
In high-stakes environments, the precision of behavioral biometrics is vital for identifying anomalous behavior, even when correct credentials are presented. Ensar Seker, CISO at SOCRadar, emphasizes that modern platforms can evaluate temporal features like typing speed and hesitation to generate risk scores without disrupting legitimate users. This progressive approach diminishes the occurrence of false positives often associated with rigid rule-based systems.
However, the rapid evolution of AI also poses a risk as cyber adversaries utilize similar machine learning techniques to create sophisticated spoofing attacks. Keeper Security suggests that adversarial testing can simulate deepfake-type threats to behavioral patterns. London highlights that these techniques can generate synthetic user sessions that closely resemble legitimate behavior while subtly integrating fraudulent elements across various biometric indicators. Training on these simulations helps organizations identify and address vulnerabilities before they can be exploited.
Traditional authentication methods are gradually being replaced by dynamic, ongoing risk evaluations that contextualize user interactions. Seker remarks, “Behavioral biometrics enables continuous, passive authentication that constantly assesses whether the current session aligns with known user behavior.” This advanced monitoring is crucial for sectors like banking and healthcare, where unauthorized access can lead to significant financial and reputational damage.
Moving forward, the next generation of behavioral biometrics will need to incorporate multi-modal signals—ranging from device intelligence to transaction history—while ensuring adherence to privacy regulations like GDPR and CCPA. The challenge lies in embedding these behavior-driven signals within existing identity and access management frameworks without causing bottlenecks or lack of transparency. London warns that while integrating behavior-driven insights can fortify security architectures, it must meet accepted performance and explainability standards to avoid introducing new vulnerabilities.
As the landscape of cybersecurity continues to evolve, finance leaders are focusing on not just thwarting unauthorized access but measuring success through concrete metrics, like the reduction of account takeovers and lower false positive rates. With more organizations adopting AI analytics to establish dynamic baselines for these performance metrics, the capacity for continuous monitoring and rapid adaptation to new threats is becoming essential in maintaining robust security protocols.
Combining behavioral biometrics with adversarially trained AI not only fortifies defenses against both human and synthetic impostors but also enhances efforts in anti-money laundering. This integration can provide richer insights for detecting fraudulent accounts or transactions. Successful implementation will depend on a delicate balance of data privacy, explicit consent, and transparent auditing processes to maintain user trust while providing high-fidelity user profiles.